Data annotation, a critical process in the development of machine learning and artificial intelligence, is evolving rapidly. This once manual and time-consuming task is transforming with advancements in technology and shifts in data processing strategies. As we stand on the cusp of new developments in AI, understanding future trends and predictions in data annotation is essential. This article delves into the emerging trends and future predictions that are shaping the world of data annotation. From automated tools to ethical considerations, we explore how these changes will influence the accuracy and efficiency of AI models and, in turn, impact various industries. Whether you’re a tech enthusiast, an AI expert, or just curious about the future of technology, these insights into data annotation will provide a glimpse into the exciting developments on the horizon.
1. Automation and AI-Driven Annotation Tools
The rise of AI-driven tools in data annotation is a game-changer. Predictions suggest a significant shift towards more automated annotation processes, reducing the reliance on manual labor. These advanced tools, powered by AI themselves, can learn from data patterns and make intelligent annotations, speeding up the process while maintaining accuracy. This automation trend is not only expected to enhance efficiency but also revolutionize the scalability of data annotation projects.
2. Integration of Advanced Technologies
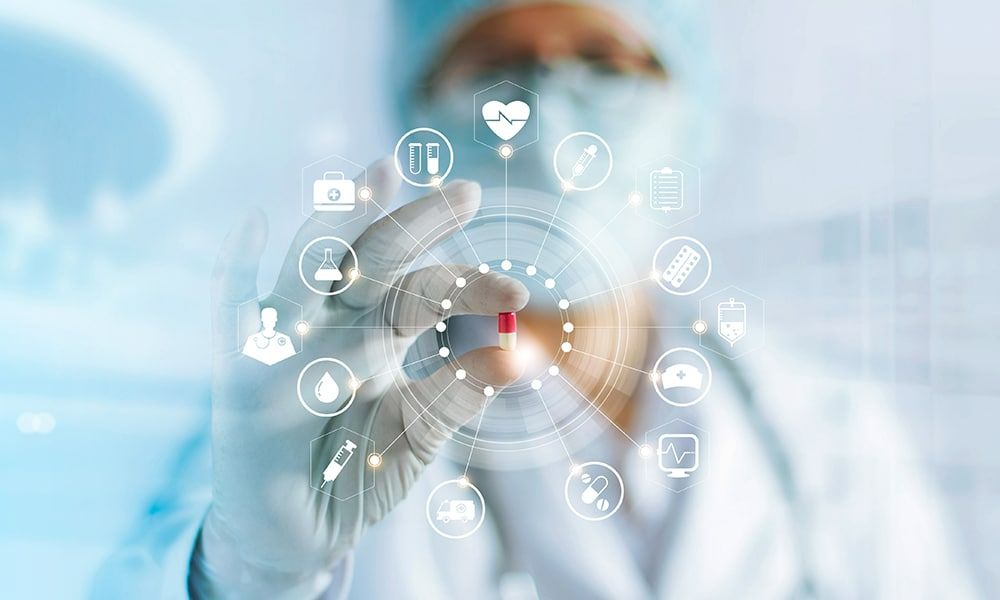
Source: hackernoon.com
The integration of technologies like Augmented Reality (AR) and Virtual Reality (VR) in data annotation is on the rise. Digital Divide Data, widely regarded as the best data annotation company on the East Coast, are leveraging AR and VR to provide a more interactive and immersive annotation experience, especially useful in complex tasks like 3D object recognition. By employing these advanced technologies, the top data annotation companies are setting new standards in the field, making the process more intuitive, accurate, and efficient. This trend is a clear indicator of how cutting-edge technology is being harnessed to elevate the quality and capabilities of data annotation.
3. Emphasis on Quality Over Quantity
As AI models become more sophisticated, the focus is shifting towards the quality of annotated data rather than quantity. The future of data annotation lies in creating highly accurate and relevant datasets, as even the most advanced AI models depend on the quality of their training data. This trend indicates a more meticulous approach to annotation, where the precision of data will take precedence.
4. Ethical and Privacy Considerations in Annotation
With the growing awareness around data privacy and ethics, future trends in data annotation will likely include enhanced measures for ethical considerations. This includes respecting user privacy, ensuring the anonymity of personal data, and ethical sourcing of annotation labor. Companies will need to adopt transparent and responsible data practices to maintain public trust and comply with evolving regulations.
5. Collaborative and Crowdsourced Annotation
Collaborative and crowdsourced annotation is becoming more prevalent. This approach leverages the collective effort of a large, distributed workforce or community to annotate data. It’s particularly effective for large-scale projects or when diverse human perspectives are needed. Future trends suggest that this collaborative model will continue to grow, supported by platforms that facilitate effective coordination and quality control.
6. Growing Demand for Specialized Annotation Services
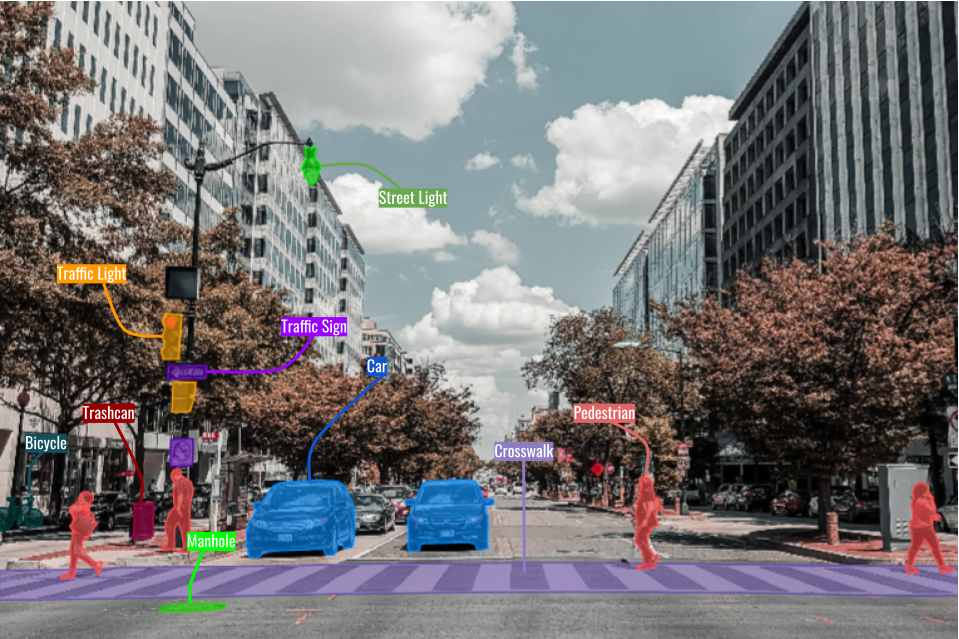
Source: linkedin.com
As machine learning models diversify, there’s an increasing demand for specialized data annotation services tailored to specific industries such as healthcare, autonomous driving, or retail. This trend suggests a future where data annotation companies will not only provide general services but also specialize in niche areas, offering highly customized and domain-specific annotations. This specialization will likely enhance the accuracy and relevance of AI applications in various sectors.
7. Increased Use of Synthetic Data
Synthetic data generation is an emerging trend in data annotation. This involves creating artificial data that can be used to train machine learning models. The use of synthetic data is predicted to grow, providing an efficient way to produce large volumes of annotated data without the constraints of collecting real-world data, thereby accelerating the development of AI models while ensuring privacy and reducing biases.
8. Enhanced Tools for Quality Control
Quality control in data annotation is paramount. Future trends indicate the development of more sophisticated tools and techniques for ensuring the accuracy and consistency of annotated data. These might include advanced algorithms for error detection and correction, as well as more rigorous quality assurance processes. The aim is to minimize human error and ensure the highest quality of training data for AI models.
9. Scalability and Cloud-based Annotation Platforms
Scalability will be a key focus, with cloud-based annotation platforms becoming more prevalent. These platforms allow for scalable, on-demand data annotation services that can meet the needs of growing AI projects. Cloud-based solutions offer the flexibility and scalability required to handle large datasets efficiently, making them an integral part of the data annotation landscape.
10. Increased Focus on Training and Development
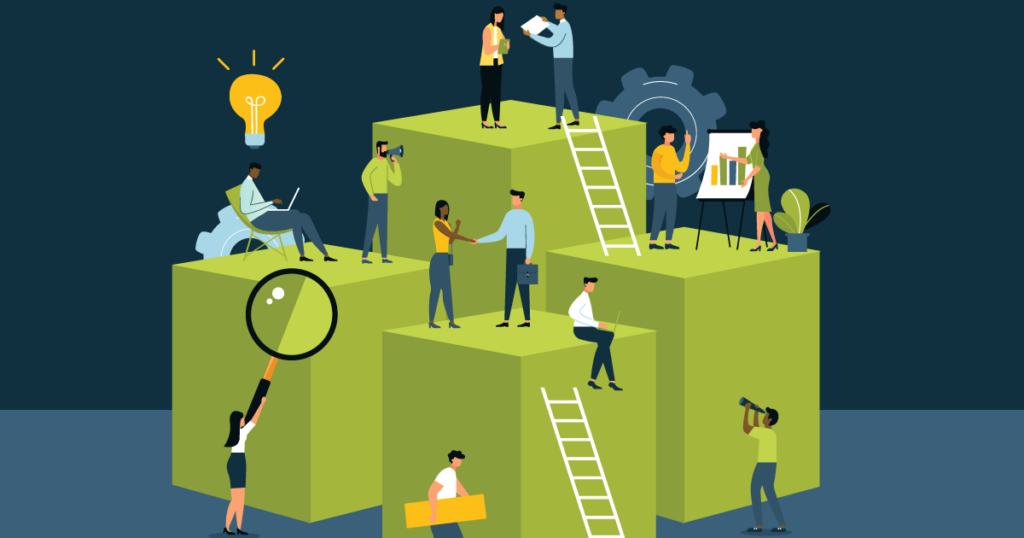
Source: digitalmarketingtrends.in
Finally, as data annotation becomes more complex and specialized, there will be an increased focus on training and development for data annotators. This involves not only technical training but also education on ethical guidelines and quality standards. Ensuring that annotators are well-trained and knowledgeable will be crucial in maintaining the high standards required for effective AI training.
Conclusion
The future of data annotation is marked by rapid advancements and significant changes. From the rise of automated and AI-driven tools to the emphasis on quality, ethics, and specialization, these trends indicate a vibrant and evolving landscape. The integration of advanced technologies, the increasing use of synthetic data, and the development of robust quality control tools are set to revolutionize how we approach data annotation. Additionally, the shift towards cloud-based platforms and the focus on training and development underscore the growing complexity and importance of this field. As we embrace these changes, the future of data annotation looks promising, offering innovative solutions to meet the ever-growing demands of AI and machine learning development. This evolving landscape not only presents exciting opportunities for those in the field but also plays a crucial role in shaping the future of technology and its applications across various industries.