When it comes to crunching and applying big data, there are a wide variety of terms in use today. Some refer to complex algorithmic programs as computer learning or computer predictions, while others opt for the simple ‘algorithm’.
Each computer learning program is tailored to tackle a specific problem or help professionals see a larger picture by investigating massive amounts of data points. Every program has a different purpose—whether that be scientific, social, or recreational.
Differences in purpose and application mean that AI programs fall under a broad umbrella of titles. In the world of trading stocks, predictive learning programs are known as ‘algorithmic trading‘. In the world of sportsbooks, users opt-in for ‘computer predictions’ that skirt by human error.
Regardless of terminology, more and more industries worldwide are turning to computer programs to help them analyze and apply complex data points. To the human eye, these figures may seem like little more than an endless array of numbers. To a foolproof algorithm, there’s another world behind statistics.
Hollywood
Finding the Next Blockbuster
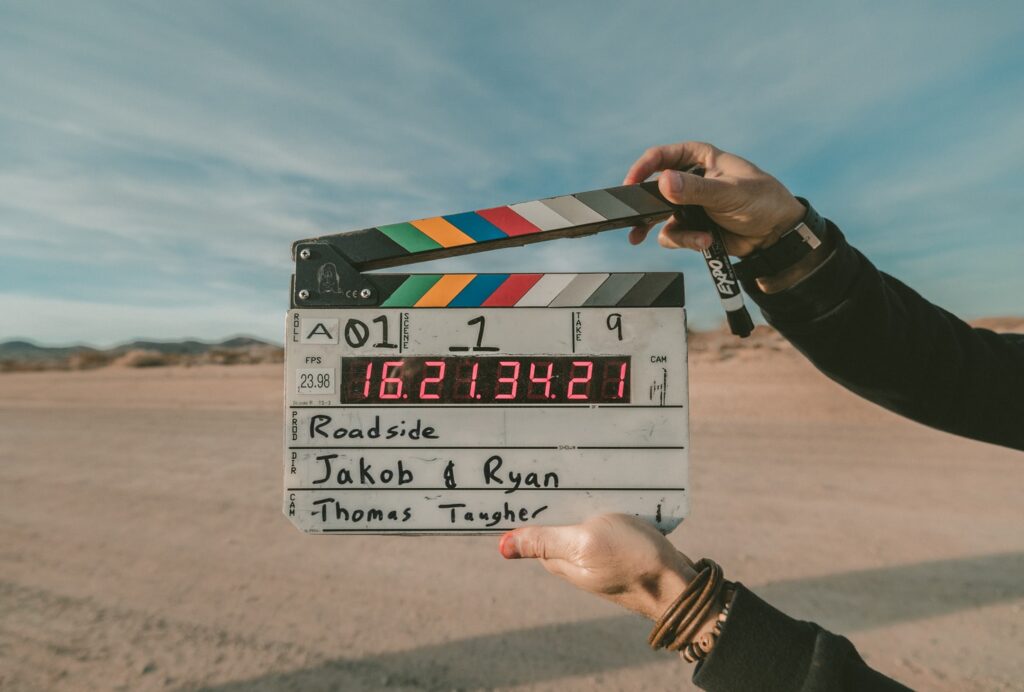
img source: unsplash.com
Hollywood’s biggest production companies aren’t afraid to go out on a limb to find the next ‘big thing’. Whether it’s harnessing an up-and-coming actor or finalizing an exciting new script, entertainment and media companies funnel billions into their next project.
Given the industry took home $2.1 trillion in 2018, according to Outlook, funding an algorithm to help predict the next blockbuster is pocket change. In this case, computer learning programs study the conditions surrounding mega-hits, like the Avengers franchise.
In 2018, global spending on entertainment and media AI programs reached $950 million, according to Research and Markets. However, not everyone is sold on the industry’s love of algorithms. Leading entertainers and certain producers have vilified computer learning for decreasing the diversity of cinema in Hollywood.
Sportsbooks
Compiling Centuries of Data
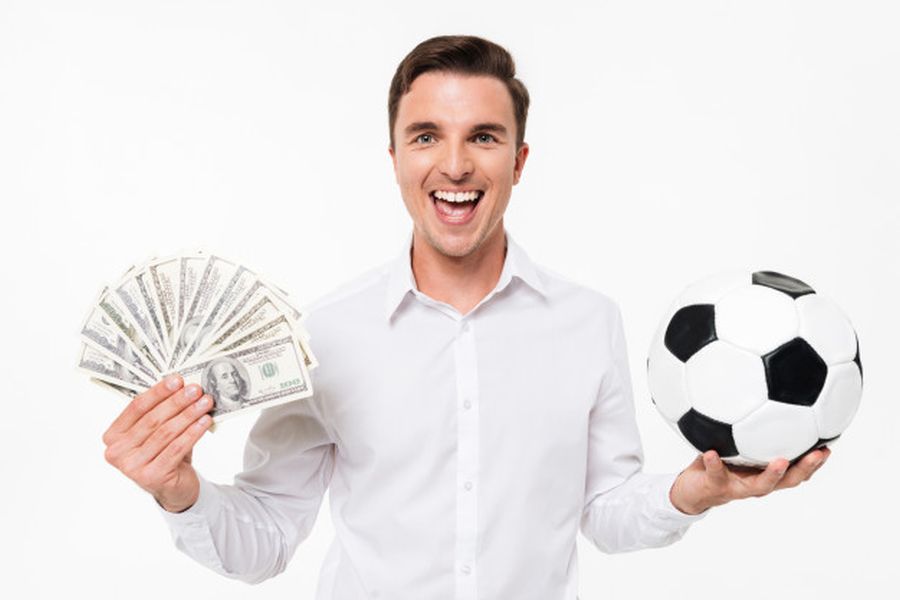
img source: unsplash.com
As one of the more recent tools utilized by sportsbooks, specialized algorithms handle copious amounts of data to make predictions about sporting events. In most cases, predictive algorithms sort through data from the start of major league sports. For context, the NFL began in 1920, the NBA in 1946, the MLB in 1869, and the NHL in 1917.
This means top computer predictions aren’t just looking at Mike Trout of the LA Angels—they’re also cross-referencing Trout’s stats against the team’s record from their inaugural season in 1892. Despite the growing relevance of big data for oddsmakers, sports analysts and pundits still have a place at the table alongside their trusty algorithms.
For example, a FanDuel review from OddsChecker highlights offerings like welcome bonuses and an app review for the DFS and sportsbook. However, the US’s most popular sportsbook also utilizes computer prediction capabilities to cater to data-driven sports fans—as well as spearhead the future of sports wagering.
For the time being, sports analysts will continue to inform how algorithms apply data. But moving forward, it’s possible computer predictions will be doing more than setting the outcome of a sports event. They’ll also be balancing point spreads and setting over/under limits.
Organic Biology
Compiling Experimental Analysis
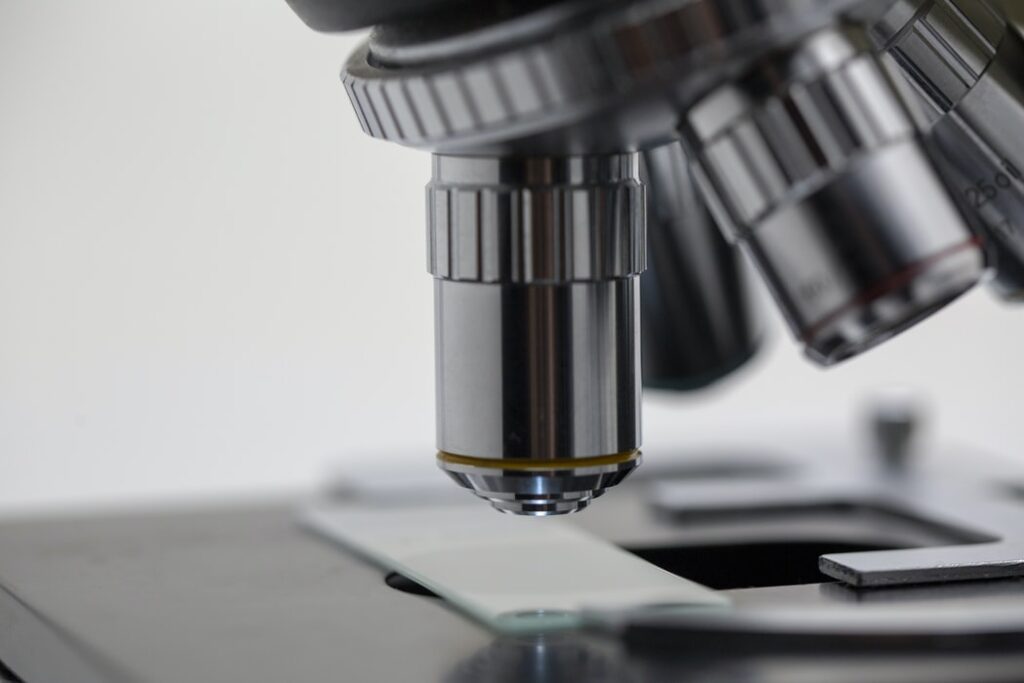
img source: unsplash.com
One of the most data-intense areas of organic study revolves around the human genome and the neural network. Both areas of organic biology require vast sequencing of data points derived from molecular DNA to the brain.
Not only are computer programs used to track and record data from both fields of biology, but algorithms are also used to replicate scientific conditions. This means biologists can experiment and study with algorithms rather than people, as these programs imitate the human body.
At the moment, top applications include predicting illness or forecasting illness in patients with neural disorders, as well as assessing if and how genes are passed on to the next generation. From there, scientists are able to further refine and cater to studies conducted on people.
Stock Markets
Forecasting New Markets
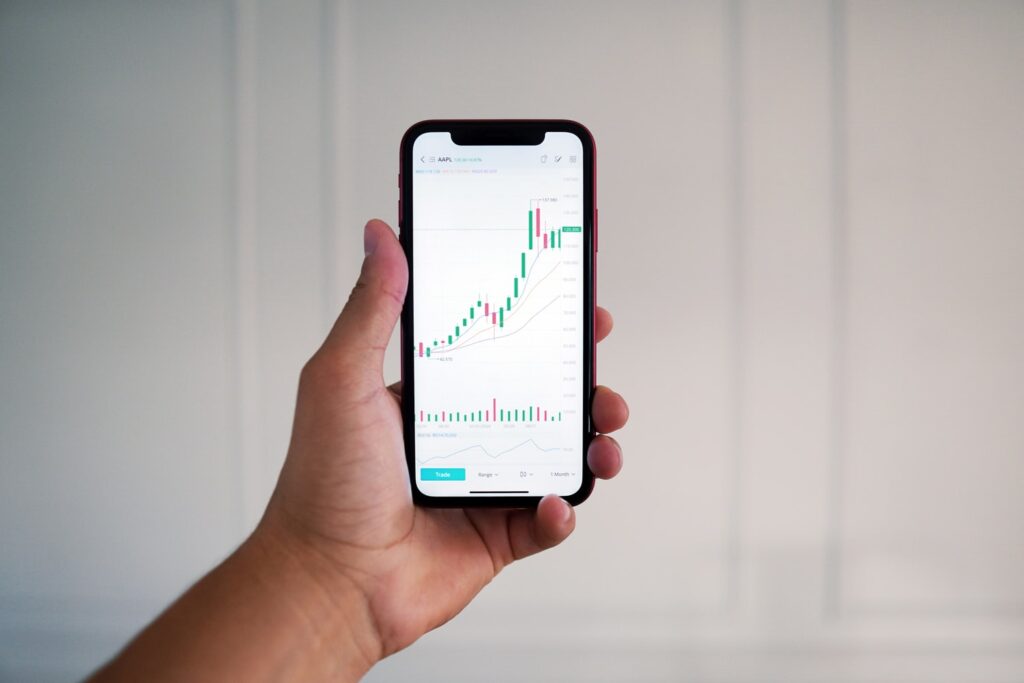
img source: unsplash.com
According to Experfy, about 70 percent of all trades in the US stock market are now handled through algorithmic trading. In other words, Wall Street is inundated with bots, which help foster fiscal efficiency without compromising future innovation.
Algorithms are highly effective at predicting new markets for top investors. Financial technology professionals dictate the conditions around which certain stocks should be bought and then sold. From there, bots are able to deliver high-frequency trading to capitalize on market shifts, buying and selling shares at an unfathomable speed.
Already, test-phase environments have been created that help professionals try out their algorithms before going live at the Stock Exchange. In terms of the automation of entire industries, predictive algorithms related to fintech will soon be able to handle real-time decisions based on previous machine-learning.
Social Media
Personalizing ads
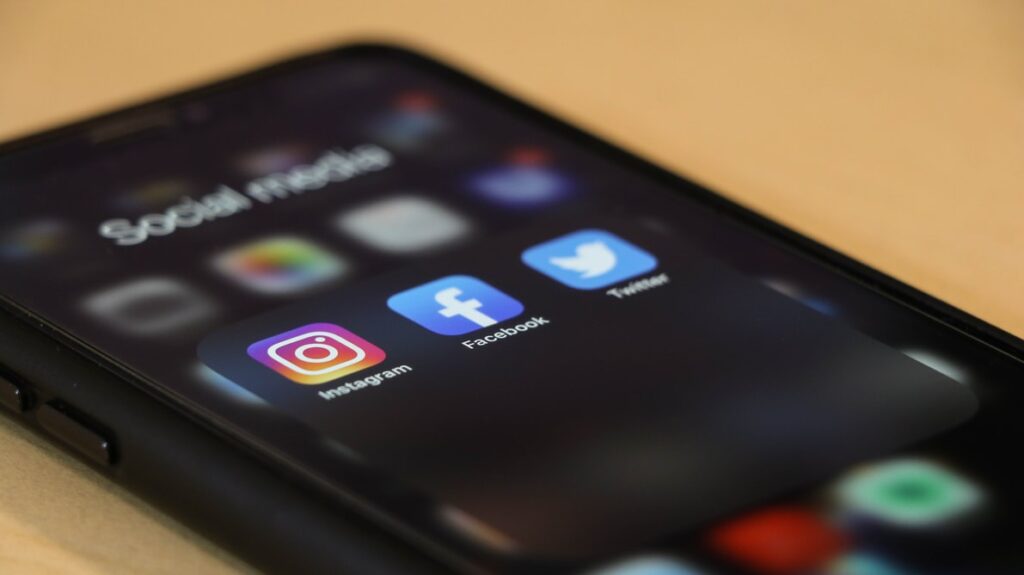
img source: unsplash.com
In recent years, the mega-popular social media platform, Facebook, came under fire for collecting and selling data points from its users. Though the presence of a bot on the feeds of hundreds of millions of users wasn’t shocking for most digital natives, the diversity with which these data points could be applied stunned many.
On the average social media page, a variety of predictive algorithms are working overtime to deliver multiple functions. One of the primary goals is to ascertain which products or services a user will be sympathetic toward in order to drive sales toward companies that pay for advertising. This connects users to products and services they may be interested in while driving more advertising money to the platform.
But the world of social media algorithms goes much deeper. Certain platforms allow for live location sharing, which means a computer learning program can cross-reference a user’s location to nearby shops and further cater ads on their feed to reflect what they’ve likely seen and done that day.
Additionally, the feed itself is fully informed by algorithms that decide what content each user is most likely to like, share, or comment on. This means that content that doesn’t meet standards of the frequency of engagement and previous interaction is likely swept from a user’s feed.
On one hand, these algorithms connect users to the content they like and enjoy, erasing the need to curate a feed. On the other hand, predicting what a user wants to see can create a false ‘bubble’ that eliminates the diversity of posts.